Comparing and Combining Curriculum Learning and Behaviour Cloning to train First-person Shooter Agents
Research
- The project
-
This research was my master thesis. During my systematic review, I found that there was little to no research on comparing or combining curriculum learning and behaviour cloning to train agents for first person shooter games, and decided to use this as a basis for my thesis.
-
With the advancements in ML and Neural Networks, it is now possible to teach an agent to perform a task. By using Unity’s ML-agents toolkit, we now have the tools inside the game’s engine to teach and train our own AI agents, as opposed to programming their behaviours. With these tools we can not only better research ML, but we can much more easily implement them in commercial projects, bringing ML to the consumer.
The main motivation for this dissertation is to use these new tools to create better and more enjoyable AI opponents for players. We want to evaluate whether it is possible to create smarter and more challenging opponents through ML and RL in games. - Aim, objectives and question
-
The aim of this research was to further research RL applied to FPS games, with that in mind, I proposed to the following research questions:
- Can Behaviour Cloning yield trained agents with better performance than Curriculum Learning in FPS games?
- Does combining Behaviour Cloning and Curriculum Learning bring better performance in the creation of bots for FPS games?
The main objective was to compare the Curriculum Learning and Behaviour Cloning training architectures and see not only which one brings better results, but also if combining the two is feasible and can bring better results than just using one of them. - Development
-
Over the course of several months, I used unity to create a project with the ML-agents toolkit. I created a curriculum training method and a behaviour cloning one and trained 2 teams of 3 agents for each method.
After the curriculum training and behaviour cloning agents were finished, I created another 2 teams of 2 different methods of combining curriculum learning and behaviour cloning.
For this research, I opted to make agents that use ingame variables as opposed to feeding a camera to to neural network. I decided on this because I wanted to create more lightweight agents, since I was working with just my personal hardware.
The agents simulated sound by sending signals to each other’s on certain actions, and simulated sight by raycast and comparing angles.
The environment was very simple. being just a square with 4 boxes serving as obstacles. Using a more complex environment would only increase training time and deviate from the objective of the research.
To speed up training, I used a parallel training setup with 6 environments, meaning that there were 6 copies of the same environment running simultaneously. - Hardware used
- CPU: Intel core i7-7700HQ @ 2.80GHz
- RAM: 16.0 GB
- GPU: NVIDIA GeForce 1050 @ 4GB VRAM
- Training and testing
- To test which training architecture achieves the best results, I trained several agents to play a simple deathmatch FPS game, where the agents face each other in square shaped 1v1 arenas with four obstacles. I trained one team of three agents for each one of the two training architectures, plus another two teams, also of three agents, for the combination of Curriculum Learning and Behaviour Cloning, with each one of these teams using different Behaviour Cloning data. Each agent was trained for one hundred million steps.
- Agents
-
Agents receive input in the form of variable input only. I developed a rudimentary way to simulate sound and sight so that the agents know the opponent’s location from simulated sound or by rotating to face them.
The agents navigate the environment with the help of the sensors provided by the Unity ML-agents toolkit, these sensors raycast in various directions, telling the agent where obstacles are. - Agent Sound
- While moving, spawning and with every shooting action, the location of the agent or target will be transmitted to the other agents in the environment. the agents are inputted the latest received “sound”, there is no limit on distance and this data is transmitted to the other regardless of position.
- Agent sight
- Agents will raycast each step to the opponent. If the raycast succeeds, then the angle of the impact vector will be compared to the agent’s forward vector. If the angle is less than 45º, then the enemy is being seen by the agent giving it a set reward. If the angle is less than 15º, the reward is increased.
- Weapon
- The agent’s weapon is a hit scan weapon with infinite ammo that can shoot once every five hundred milliseconds, meaning that after shooting, the shooter must wait five hundred milliseconds before shooting it again. Each shot does twenty-five damage, meaning that, the targets, and agents, who all have one hundred health, are destroyed in four shots.
- Inputs (observations)
-
The agents have a total of seven observation inputs, totalling at twelve float values.
- Agent Position (3 floats) – The agent’s current 3D coordinates
- Agent’s Rotation in degrees (1 float) – The agent’s current Y Euler angle.
- Last seen Enemy Position (3 floats) – the 3D coordinates of the last seen enemy
- Last non-self-made sound Position (3 floats) – the last sound heard that was not produced by this agent.
- Can shoot (1 float) – if weapon is ready to fire.
- Current Health difference to max health (1 float)
Besides these, the agent also receives inputs from the 3D ray perception sensors, which are sensors included in Unity’s ML-agents toolkits. - Curriculum Learning Training
-
The Curriculum Learning was split into eight phases. I distributed the tasks between them, making each phase harder than the previous, the objective was to teach the agents the various things they needed to learn such as enemy detection, aiming and navigation.
During the various phases, the agents find three types of targets, and then at the very end it fights against itself. - Behaviour Cloning Training
-
To train the Agents using Behaviour Cloning, we first recorded one hundred wins in Curriculum Learning’s phase eight, which outputted a demonstration file that was inputted into the agents via the configuration file. To train the agents with Behaviour Cloning, I used GAIL, one of types of imitation learning available in ML-Agents.
The training setup of the Behaviour Cloning agents is the exact same as phase 8 of the curriculum learning. The agents started with just the knowledge they developed from the demo and each one of the three agents trained against their own clone for one hundred million steps each. - Combination of Curriculum Learning and Behaviour Cloning.
-
To train the agents using the combination of Curriculum Learning and Behaviour cloning, the agents were inputted a previously recorded demo file via configuration file, and then started training from phase one of the Curriculum Learning.
Two sets of teams of three agents were created as I tried to find an effective way to combine the two architectures. I called these teams the “Duel-type combination” and “Basics-type Combination”. - Duel-Type Combination
- In this combination type, the demo file used in the Behaviour Cloning method was inputted to the agents via the configuration hyper parameters. A Team of three Duel-type agents were trained for one hundred million steps each.
- Basics-Type Combination
-
For this attempt, we recorded a new demo file, this time with fifty episodes throughout the Curriculum Learning method’s stage one through four. We then inputted this file to the agents via the configuration hyper parameters.
For both types, after the demo files were input, they started training from phase one of the Curriculum Learning, trying to clear each stage to reach the final eight stage. Each one of the three agents trained for one hundred million steps. - Hyper parameters
-
The used Hyper parameters config file are almost the same for each of the training architectures, the only difference is that during the Behaviour Cloning and combination training, the reward signal is changed from “extrinsic” to “Gail”, and the behaviour demo file is added.
Bellow you can see the various hyper parameters used:
- batch_size: 1024
- buffer_size: 10240
- learning_rate: 3.0e-4
- learning_rate_schedule: linear
- beta: 1.0e-2
- epsilon: 0.2
- lambd: 0.99
- num_epoch: 3
- normalize: false
- hidden_units: 128
- num_layers: 2
- extrinsic:
- gamma: 0.99
- strength: 1
- max_steps: 100000000
- time_horizon: 64
- summary_freq: 5000
- The testing System / Taking Results
-
To test the agents against each other’s I made each battle against all the different types of agents five times each. To do this I devised a matchmaking system that picks an agent, then makes them battle each agent of the opposing type until they have accumulated either five successful battles or ten timeouts against that agent. Note that two agents of same type will never battle each other’s (example: Agent trained in Curriculum Learning vs Agent trained in Curriculum Learning), as I wanted to compare the different training architectures.
The battles use the same exact mechanics as phase eight of the of the Curriculum Learning program. Meaning that each participant spawns opposite of each other, and then must destroy their opponent two times to achieve victory. Because the agent are not perfect players, I implemented a timeout system for each battle. After a battle starts, the agents have two minutes to destroy their opponent before the battle resets. after an agent scores a kill, the timer is reset, and they once again have two minutes before the battle resets. this system is in place to prevent cases where the agents never find their opponents and are stuck during large amounts of time. - Total number of battles won.
- Total number of battles lost.
- Total number of successful battles
- Total number of battles that timed out.
- Total number of battles
- Total number of shots missed.
- Total number of shots hit.
- Total number of enemies destroyed.
- Total number of times destroyed.
- I ran this testing environment twice, once with obstacles enabled and once without because I wanted to evaluate with and without the need for map navigation and see how the results differed. In the results section I will present the results from these tests.
- Metrics and Performance Evaluation
-
Performance is measured through the parameters obtained in battle by the agents. Each parameter is a number that we use to measure the agent’s performance, with wins, successes, hits, and kills being better the more there are and losses, time outs, total games, misses, and deaths being better the less there are.
When I mention an agent have better or worse performance, I am talking about these metrics and numbers being better or worse when compared to their peers. - Table of results with Obstacles
-
Next I will present the results for the tests with obstables in form of a table with numbers for each one of the obtained metrics.
- As you can see, the curriculum agents had the best performance, achieving over 95% victory rate.The cloning agents won against the combination ones but still lost against the curriculum. Meanwhile the combination agents failed to ever achieve one win and ended up timing out when playing against each other’s. The kill to death ratio also shows that the curriculum agents outperform other by far.
- Table of results without Obstacles
-
Next I will present the results for the tests without obstables in form of a table with numbers for each one of the obtained metrics. The point of testing without obstacles was to remove the aspect of navigation and seeing if the results changed in any way.
-
The results without Obstacles are more balanced, we see that the cloning agents performed better but still lost to the curriculum ones. There were less timed out battles and more deaths in general, meaning that the agents were able to find their opponents more easily.
Overall, the performance is better, but the results remain the same, with the Curriculum Learning agents being the best, followed by the Behaviour Cloning ones and then the combination ones. - Observations
- Some things of note that are not captured by numbers must also be exposed.
- Curriculum Learning Agents
- The curriculum agents were all able to complete the whole curriculum. One thing to note is that as they learned to be constantly shooting, which lead to them having many missed shots. Other than that, there were no notable problems or anomalies.
- Behaviour Cloning Agents
- The Behaviour Cloning agents did not constantly shoot like the Curriculum Learning team. These agents were also much less aggressive than the curriculum ones and did not explore the map as much. As a result, they timed out due to not being able to find their opponents various times.
- Duel-Type Combination Agents
- The first team of combination was never able to progress beyond phase one of the curriculum. They performed very poorly in both training and in battle when compared to the Curriculum Learning and Behaviour Cloning agents.
- Basics-Type Combination Agents
- The second team of combination was able to reach phase four of the curriculum but were unable to progress any further. Just like the first combination team, they performed very poorly in battle.
- Overall
-
In general, all the teams had issues with pathfinding and often found themselves getting stuck on the obstacles. Another issue was that they were not aggressive enough and many times would just stay doing circles in the area where they spawned, trying to find the enemy.
The combination teams when pitched against each other had a very hard time finishing the match, as they either couldn’t find each other’s, or couldn’t hit each other’s. this meant that most of their matches ended in time outs. - Discussion
- Next I expose my thoughts on this whole experiment and its results
- Comparing Curriculum Learning and Behaviour Cloning
-
The first proposed question was to compare the Curriculum Learning and Behaviour Cloning training architectures and see which one yielded the best results, to investigate this I used as metrics the number of wins, kills, deaths and shots hit after we made all agents battle each other’s.
The agents trained with the Curriculum Learning method obtained the best results with and without obstacles: with the most wins, least time outs and a much better kill to death ratio, they were the ones most capable of finding and destroying their opponents.
Meanwhile the agents trained with Behaviour Cloning, although able to beat the combination agents, could not beat the Curriculum Learning ones, as the Curriculum Learning agents barely have any loses. They also exhibit many time-outs meaning that they were not aggressive enough and failed to find their opponent.
The only place where I can say that the Behaviour Cloning agents are on par with the Curriculum Learning ones is the accuracy, most likely because unlike the Curriculum Learning agents which were constantly shooting, the Behaviour Cloning agents would try to shoot only when the enemy was in their field of vision thanks to the data from the demo.
Even though without obstacles the agents could more easily find their enemies, this still did not change the outcome where the Curriculum Learning agents come out on top.
With these results, I reach the conclusion that the Curriculum Learning method that I developed achieves much better results than using Behaviour Cloning. I believe this is because the Behaviour Cloning agents become so focused on the provided demo data that they cannot develop new strategies during training, this led them to lose almost all battles against the Curriculum Learning agents who learned from zero on how to achieve the best results. - Combining Curriculum learning and Behaviour cloning
-
The second proposed question was about combining the training architectures of the Curriculum Learning and Behaviour Cloning and seeing whether this combination was viable and created a significant improvement in the agent’s performance. Once again, the metrics are the exact same as in the previous question. For this we tried two methods of combination:
The first method never managed to go beyond the first Curriculum Stage, failing to ever destroy the very first immobile target, and while the second method was looking promising during training, it stalled and failed to go beyond the fourth stage.
Both teams failed to achieve any meaningful results, having both failed to even reach the end of the Curriculum Learning program during training. Even with GAIL, the agents relied too much on the provided demos and failed to adapt to any difference in the environment.
During testing they were repeatedly destroyed by their opponents, and when facing each other’s they almost always ended the battle in a time out. Looking at the concrete results we see that these agents were a complete failure, with the second method not achieving one single win while the second method only managed to win three matches. Almost all their success matches ended in defeat, and even their hit percentages are abysmal compared to the Curriculum Learning and Behaviour Cloning agents.
With these results I conclude that Curriculum Learning and Behaviour Cloning are incompatible, as the demonstration data interferes with the curriculum’s progression system.
The explanation for these results seems to be that the agents are so focused on the data created by the demo that they fail to adapt to any environment that is not the exact same as the one from the demo. The second method saw some success in reaching the fourth stage of the curriculum because the environment was only very slightly different from the provided demo, but the moment the target started moving, the agent could no longer aim at it and failed to progress any further.
Phase | Purpose |
1 | Teach the agent to look and shoot at the enemy. |
2 | Teach the agent to aim at an enemy in front of them. |
3 | Teach the agent to look around for the enemy. |
4 | Teach the agent to aim at a moving enemy. |
5 | First Step in Teaching navigation to the agent. |
6 | Second step in teaching navigation to the agent, also forces the agent to train how to search for an enemy that has respawned. |
7 | Third step in teaching navigation. |
8 | Live battling. |
To get the results, I saved the following parameters from the battles per agent:
Team | Av. win % | av. Successes % | Av. Time Out % | Av. Hit % | Av. Kill/Death Ratio |
---|---|---|---|---|---|
Curriculum | 95.33 | 71.1 | 28.9 | 5.78 | 11.02 |
Cloning | 54 | 24.8 | 75.2 | 5.98 | 1.79 |
Combination1 | 0 | 21.3 | 78.7 | 0.63 | 0.05 |
Combination2 | 0 | 17.6 | 82.4 | 0.34 | 0.01 |
Team | Av. win % | av. Successes % | Av. Time Out % | Av. Hit % | Av. Kill/Death Ratio |
---|---|---|---|---|---|
Curriculum | 92.67 | 91.3 | 8.7 | 9.25 | 6.19 |
Cloning | 69 | 42.8 | 57.2 | 8.85 | 2.61 |
Combination1 | 2.33 | 23.8 | 76.2 | 0.94 | 0.13 |
Combination2 | 0 | 31.7 | 68.3 | 0.75 | 0 |
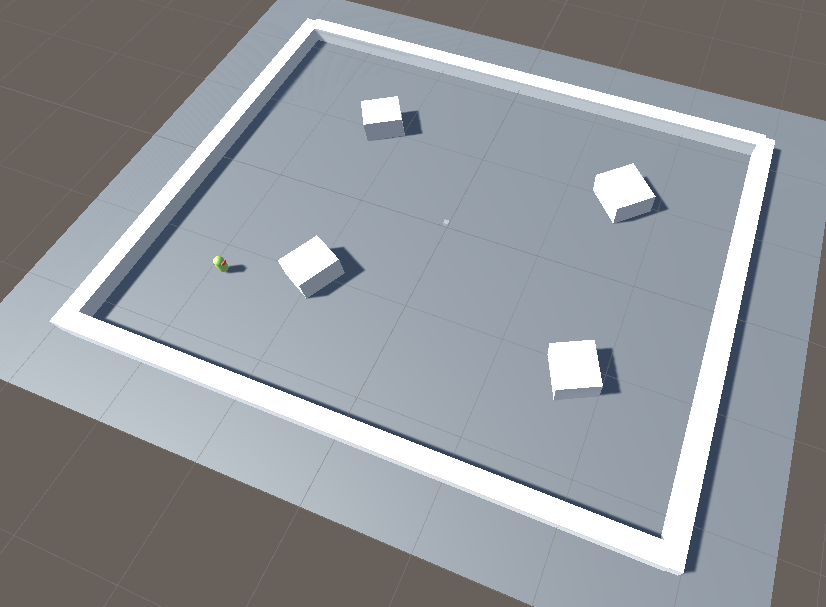
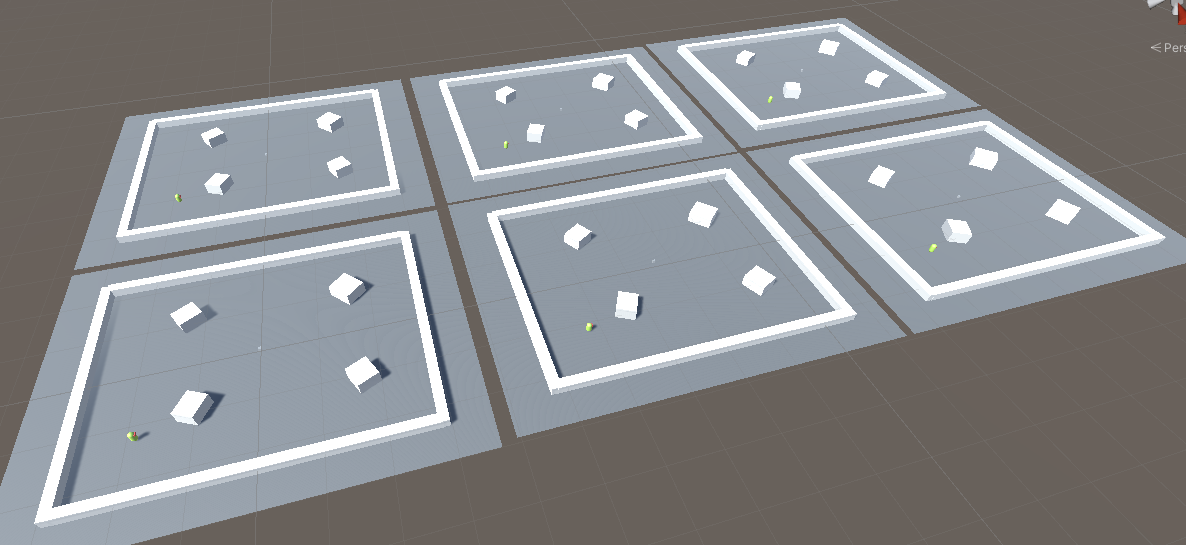
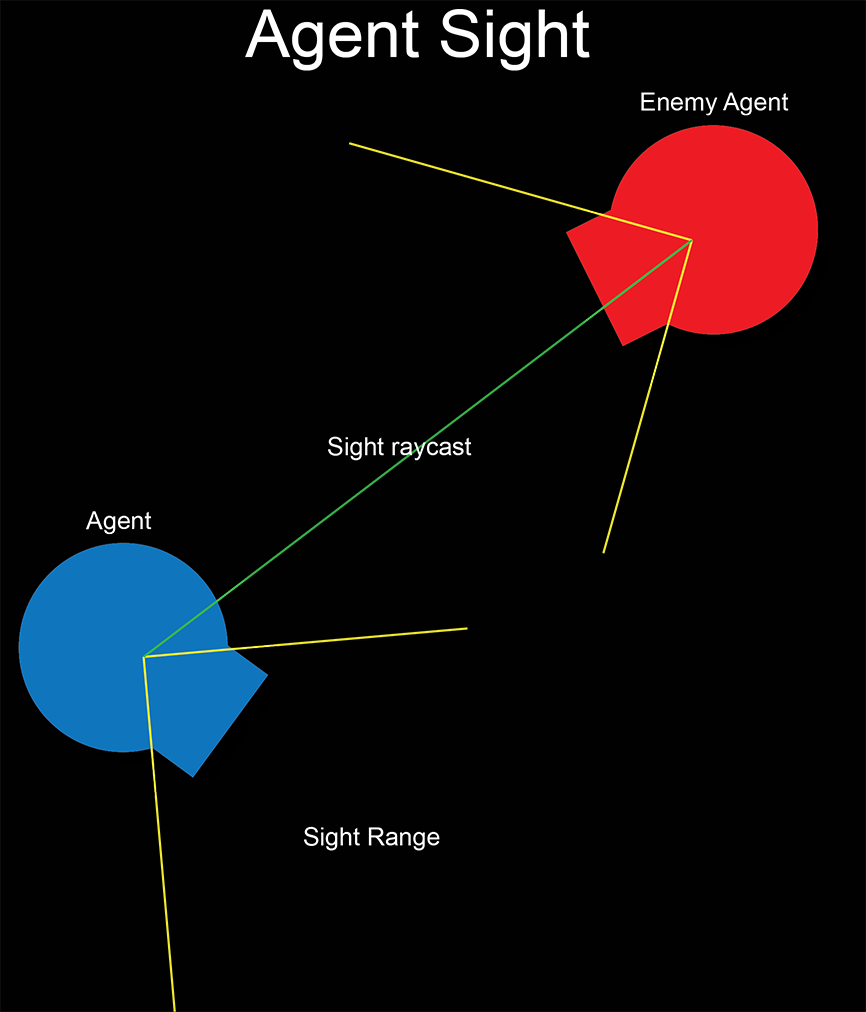
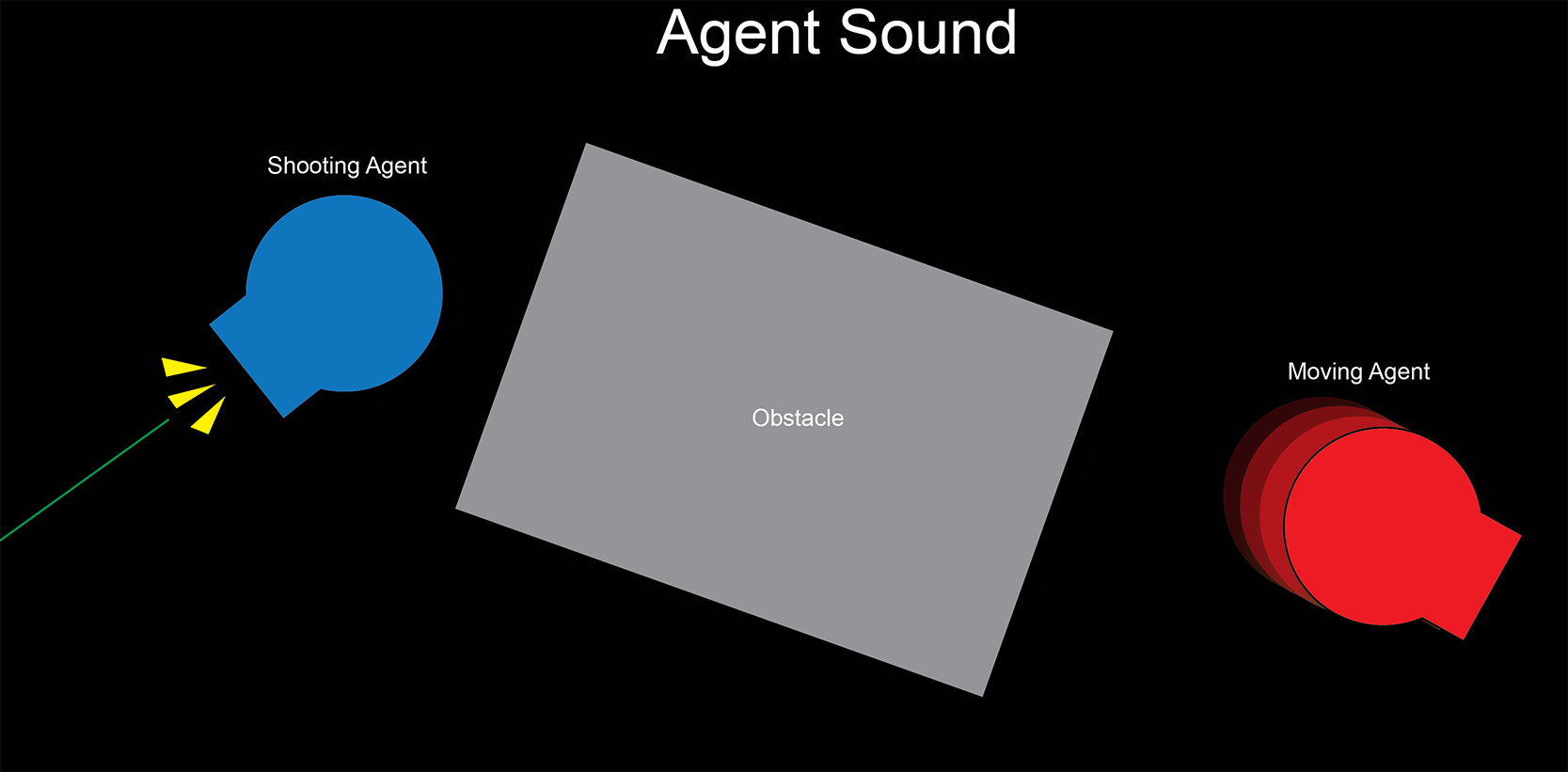